Jump to section
Everything local authorities do depends on data and this includes designing and stimulating smart regions, with a range of public and private sector partners. Councils are increasingly creating data and service hubs, pooling data from multiple sources, linking it, sharing it and analysing it for policy insight and planning, as well as service delivery and management.
Local authority services play a central role in the development of ‘smart places’, and the data needs that support these developments are often complex and sensitive, especially in in areas such as:
- Support for vulnerable children and adults
- Ensuring that traffic is free-flowing whilst tackling pollution and environmental pressures
- Helping a wide range of public protection agencies run smoothly and efficiently
- Supporting jobs and the local economy to prosper, whether in a rural or urban area.
In the many case studies, there is an emerging pattern of technologies to support rural and urban infrastructures, such as IoT, being used to improve public service outcomes – to reduce pollution, to keep people safer, to improve efficiency of infrastructures.
A growing range of apps are available, some developed by councils, often using open data, particularly for travel planning, but also for a wider range of public services in an area, such as coordinating everything from bin collection and council tax to benefits and social care.
Socitm has already undertaken considerable research into the topic of smart places – find all our publications in the resource hub.
Community information hubs
As local authorities increasingly become brokers of services, collaborating, undertaking joint procurement and facilitating the connection between related services, so they need a mature approach to data management and sharing. That is the purpose of this collection.
More detail about the changing role of local authorities as aggregators of services, their role in support for smart places and their responsibilities in a wider community cyber protection can be found a number of recent Socitm research publications. The following diagram is designed to help a local authority the context for a wider community data strategy.
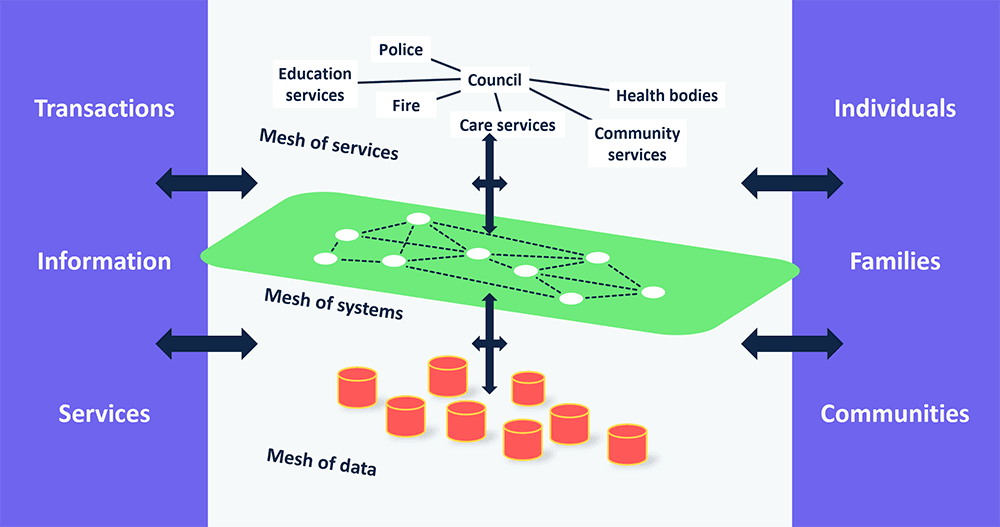
There is widespread agreement from this research that identifying and starting with a small number of key data sets can allow intelligent linkages to be made by public bodies.
There is also widespread concern expressed by local authorities found in this research about restrictions on sharing personal data – for example, refusal to allow the NHS number in social care invoicing. That challenge here lies in national government legislation to ensure true integration has a data level between NHS and social care, including changing culture of trust between social care and NHS.
There is also a view from some that IT is part of the problem, with outdated processes for data management and systems development, as well as a heavy focus on the technology rather than the data.
Data maturity – self assessment
It is not easy to objectively judge where you are in terms of organisational data maturity. But trying to rationalise how mature you are does have big benefits in planning ahead and knowing where to start on a data project. There is also a range of tools out there to help you to check your organisation’s data maturity in specific areas, such as data quality, or cyber awareness.
Data maturity is more than having a recognition of the importance of data and making some investments to manage and to safeguard it. Sometimes it takes a data incident to drive wider appreciation of data value and risk, and how these need to be better managed.
In assessing data maturity it is important to consider more than the views of the data specialists, or even executive and service leaders. Asking front line staff confidentially and anonymously how they view the organisation’s maturity of data management can be more illuminating, especially since it is ultimately staff who need to ensure high levels of data control and protection, as well as exploitation.
Data maturity checklist example
Q1. Do you know where all your data is, especially personal data?
Immature example: We know where some of it is, because it is in managed systems, and we have undertaken a GDPR audit. But there is much data we have that we don’t realistically know about – unstructured, old and archived or just hidden (‘dark data’).
Mature example: All our data is classified with a clear data owner. The policies and processes are regularly audited, and we learn from any information ‘incident’. We have methods to manage structured and unstructured data and are confident there is no hidden data held in paper of electronic format that we cannot expose and classify.
Q2. Are you GDPR compliant?
Immature example: We don’t know fully. We have undertaken training and awareness, and we do have the necessary policies in place. We have also identified most data that contains personal information.
Mature example: We set up a detailed GDPR compliance project to meet the deadline and all our data was sorted and classified, with the supporting policies and procedures. Since then we run tests and audits routinely and we are confident we can deal with any subject access request quickly and efficiently.
Q3. Do you have a clear data sharing policy and procedure?
Immature example: Yes, in key areas where sensitive data is involved, and we do encrypt data. However, we don’t share a great deal and the methods of sharing are convoluted and difficult.
Mature example: Yes, in all areas. This includes ensuring all our suppliers and partners comply and we use the NCSC and Socitm data handling guidance in all areas.
Q4. Are the main board and political leaders encouraging a data-oriented culture?
Immature example: To an extent – there is awareness of the risk and support for the importance of data security and careful data handling. But most would not say they are information experts or that they fully understand the complexity of data in a digital operating model.
Mature example: Yes. All politicians receive mandatory induction in information risks and data handling and are supported in their roles. Key members sponsor and champion our data sharing projects, and the main board would consider themselves to be information professionals as much as services leaders. Data is a board level topic, covered in both cyber risks assessment as well as information exploitation opportunities. Data is seen as an organisational asset not owned by individual departments.
Q5. Do you have a Chief Data Officer and are you investing in data scientists?
Immature example: Not as such, but we do have a qualified DPO and effective SIRO. There are also data specialists in key areas of the business such as social care and planning.
Mature example: Yes, and data management is not a separate professional discipline in the organisation. We are investing in data skills in all areas, and especially in a core team with a senior data lead reporting at board level. The DPO, SIRO and CIO work together with the CEO and service leaders to ensure a whole-organisation approach to data management.
Q6. Have you successfully undertaken multiagency data projects?
Immature example: No. We do share data, but it is limited, and most data projects are system projects supporting a specific technology or service outcome.
Mature example: Yes, we have had MASH projects and routinely instigate projects to share data to improve public service outcomes in the region. We are working this way successfully with health partners in particular, using rapid prototyping and data testing with a variety of tools to ensure effective data quality, matching and management.
Q7. Do you have the tools you need for effective data management and use?
Immature example: Partly – we have some good IT systems that give our professional teams the data they need on the move and in the office. However, we don’t have a full set of data analysis tools such as data matching, testing, master data management, interrogation and exposure of ‘dark’ hidden data, for example.
Mature example: Yes, although it is always changing. We have a range of tools that allow us to undertake deep data matching and analytics, spanning AI engines and reporting across multiple systems at once. Our data is unlocked from proprietary systems, so we are able to link and match as necessary, to ensure timely intervention, effective information sharing and also to manage cyber risks that come from all the data we have. We also have tools for data mining, reporting, collaboration, data cleansing, data visualisation, warehousing data and predictive analytics.
Q8. Do you archive historic data?
Immature example: Yes, data that is old is archived or deleted to reduce pressure on current data storage and processing costs.
Mature example: Not necessarily. We are clear why we collect and store data and know which historic datasets can be valuable for insight, especially into trends and changes. Where we do archive data, it is carefully classified and can readily be retrieved if required, and we have tools to automate and interrogate archive data with minimal cost overhead on live systems and higher cost data storage.
Q9. How do you ensure the right people get the data they need?
Immature example: Data is generally classified by service and systems, ensuring that professional teams can get the data they need.
Mature example: There is a complex system of checks and balances that ensure the necessary data sharing and access provided against role-based security profiles and business needs. This applies not just to our own professionals teams but also the partners and supplier with whom we work and share data. Any misuse – intentional or accidental – is quickly flagged and fixed.
Q10. Have you had a data breach?
Immature example: Yes or no!… but if you have had one you might not even be aware, and you probably won’t have looked at the wider risks it might have exposed.
Mature example: Whether or not you have had a breach, you will have policies and practices in place and tested for such an event. This includes clarification of when incidents should be reported to the ICO, NCSC or the Police. Whenever there is an incident, however small, or a ‘near miss’ it is treated as a chance to learn and to strengthen protection and awareness.
Q11. Do you have an open or closed culture with respect to data security?
Immature example: Data is seen as a risk and the challenge of disentangling personal or sensitive data is difficult, So the organisation has a policy or habit of ‘closed’ data, all held securely and internally, unless there is a specific agreement to publish.
Mature example: The overriding policy is of data openness – available to all. This then informs data policy and practice of identifying and protecting all sensitive data – commercial, personal or politically sensitive. All staff understand this and the implicit and explicit responsibilities in data handling and use.
Q12. Are staff confident and competent in data management and use?
Immature example: Staff are aware that there are policies, and they see data as something that helps them to do their jobs, but not as a corporate asset with growing levels of risk and challenge.Mature example: All staff are aware of data handling guidelines, GDPR implications and their personal responsibility in data management and use. It is not just seen as the role of specialists and job performance evaluation will typically include consideration of staff competency in data use and exploitation in their jobs.